Flash and JavaScript are required for this feature.
Download the video from Internet Archive.
Video Index
-
Introduction
Introduction and adminstration. -
The Meaning of Meaning
What do we mean when we say 'Snow is white'? Does that sentence mean the same thing to everyone? -
Contracrostipunctus Revisted
Exploration of the multiple layers of meaning in this passage. -
Defining Meaning
Meaning is the relationship between an object and its referrent. -
Encoding Information
How complex phenomena can be modeled using simple equations. -
Lindenmeyer Systems
Definition (formal grammar with self-similarity) with examples (Serpinski Gasket, Koch Snowflake). Self-similarity in nature. -
Cellular Automata
The game of life and other cellular automata.
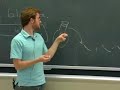
Lecture 4 video
» Download English-US transcript (PDF)
The following content is provided under a Creative Commons license. Your support will help MIT OpenCourseWare continue to offer high quality educational resources for free. To make a donation or view additional materials from hundreds of MIT courses, visit MIT OpenCourseWare at [email protected].
JUSTIN CURRY: All right. Welcome back, everybody. I'm glad to see more faces today. So the feedback looked pretty good. And once again, I encourage you guys to be brutally honest. I believe wholeheartedly in this whole idea of a democratic classroom. And having this feedback process allows to correct and make this an even better learning experience for everybody.
So I handed out a couple of things. First of all, I handed out kind of foremost chapter 10 from Douglas Hofstadter's newest book I am a Strange Loop. And that's called Godel's Quintessential Strange Loop. And I think I'm OK, and I'm not going to be sued, because as long as it's less than 10% and it's for academic purposes, it should be OK. And considering this is like 15 pages out of a 300 page book, I should be under that.
This is supplemental reading. A lot of you raised notions of like Godel numbering does not make sense. Well, it takes a lot of time to wrap your head around it. I mean, there's a reason why there was one Godel, and why he named it Godel numbering. It wasn't like it was a trivial idea. Go ahead.
AUDIENCE: Is Godel numbering sort like [INAUDIBLE] theory?
JUSTIN CURRY: So the fundamental idea behind Godel numbering is that you can take the formal patterns and any type of proving procedure, whether it's the MIU system or whether it's proving from Peano's axioms and number theory, and you can code those formal deductions into manipulations of numbers. And then, once you create this link, this isomorphism, into numbers you can then just play around with these large numbers, which then, decoded, give you back formal statements in the system you were playing with originally.
AUDIENCE: So maybe like having an equation with no solutions, almost having like an unprovable thing.
JUSTIN CURRY: Exactly. So what Latief said, it's like having an equation with no solutions is like having an unprovable thing. That's actually going to be very close to the essential idea behind Godel's proof. But if you read chapter 10, I believe Douglas Hofstadter-- out of this new handout I gave you-- does a great job of explaining some of the key ideas behind Godel's Incompleteness Theorem. And that's the main one, that systems as powerful enough as number theory are inherently incomplete.
So that's something I want to leave you at least two weeks to kind chew on, because eventually you're going to work on to chapter 9 and GEB, where he does Neumann and Godel. And that's where he proves, or does a version of Godel's Incompleteness Theorem and NGB. But It's really not as clear as I think it's done here. So that's just kind of supplemental reading.
So now, onto what I started last time and what should have been the reading assignment for today, was the location of meaning in chapter 6. I started out with this idea of the rabbit and [INAUDIBLE], but I want to scale back a little bit and really bring the question down to something which some of you will intuitively know and some of you won't have any idea. And it's the following sentence-- and please, some of you who know Spanish better, correct my spelling.
So nieve es blanca, who knows what this means?
AUDIENCE: [INAUDIBLE]
JUSTIN CURRY: OK. So everybody raises their hands and snow is white. But for those of you who didn't raise your hand, what does that sentence mean?
AUDIENCE: [INAUDIBLE]
JUSTIN CURRY: It means nothing. Why?
AUDIENCE: Because I can't relate to it.
JUSTIN CURRY: You can't relate to it. So far we've got the Latief hypothesis, which is that meaning comes from the relationship of this to yourself. And this is an idea we're going to try to explore throughout all of today's lecture. And we're going to co-teach-- Curran and I are going to do some excellent things.
So suppose you were dropped off in Mexico and just by hanging out with people you eventually learn Spanish. And you created a dictionary with a set of recursive rules. And you learned that we have certain things like, this is equivalent to is, and you understand this in your natural language.
And you create this method of breaking down sentences-- strings-- into parts, and then going to your dictionary and saying, OK, nieve es. Well, I know es is is. Nieve-- I'm not sure what that is, but my dictionary tells me it's snow. And blanca, my dictionary tells me is white.
So suppose you've dropped off in the middle of Mexico, and just by interacting with the people you've created this kind of set of recursive rules for first parsing sentences. And I think that the more languages you try to learn, the first thing you're going to discover as the stumbling block is the ability to actually parse what someone is saying. I know when I was living in Germany this past summer, basically when someone who would talk to me, it would be like, [GIBBERISH].
And I'm like, excuse me? And it didn't even sound like there were words, individually. It was just a stream of sounds. But eventually, by just being immersed in the culture, my brain ran these kind of neural network algorithms that were able to start breaking up those (GIBBERISH SOUNDS) into bier ist guht, or something like that. And you could then actually start hearing individual things and then trying to deduce the meaning of those individual parts, and then plugging them back together into your natural language into what appears to be snow is white.
But does snow is snow white mean?
AUDIENCE: [INAUDIBLE] the words themselves don't mean anything.
JUSTIN CURRY: OK. So Latief claims that maybe the words don't themselves mean anything. But what does that mean? So then the question is, suppose I wrote on a piece of paper, snow is white, and I packaged it into a bottle. So there's our scroll-- and I cork it. And I throw it into the ocean. And it drifts up on shore-- what would then be the cue?
So then suppose it lands on this island. And you see this bottle-- well, why would you suspect that the bottle meant anything? Why wouldn't you just pick up the bottle and throw it in your bag of trash as you go and try and clean up your beach?
AUDIENCE: It seems very well constructed.
JUSTIN CURRY: So Latief says it seems very well constructed. So what is it about this bottle, scroll, and cork configuration that cues you in to the idea that there's information-- that there's meaning in here.
AUDIENCE: Paper.
JUSTIN CURRY: Paper. OK. So it seems deliberate in some senses. So the idea that, well, it seems hard that accidentally some paper crept into a bottle, corked itself, and then sent it through the ocean. So Hofstadter, in this past chapter, breaks things down into three layers of meaning. He's got what's called a frame message, we've got an outer message, and then we've gotten an inner message.
So Hofstadter would argue that the framed message here is the fact that there's this strange configuration of a bottle, a cork, and a piece of paper that would cue a human being, any kind of reasonably curious human being-- and he argues that, fundamentally, curiosity is a characteristic of human beings-- that there's something to be solved here. That there's information here. That this is kind of screaming out to our stick figure on the island of [? Tombolia, ?] decode me-- figure out what I am.
So suppose then we unpack this piece of paper, and he saw this, but he doesn't speak English. How would he then proceed to try to extract a meaning from this?
AUDIENCE: You can put that into words.
JUSTIN CURRY: So Latief argues we could develop it into words. So how would we, first of all, argue that these aren't just meaningless scraps of paper-- just marks? Maybe it's like a Jackson Pollock painting or something, and it just appears to be a bunch of gibberish. Sandra or--
AUDIENCE: Aren't there repeats? Like, w, w, [INAUDIBLE].
JUSTIN CURRY: OK. So Sandra said that there's repetition. There appears to be certain things that-- there's even this concept of a space, that there should be something that's parsed here. There's separation into parts. Latief.
AUDIENCE: Maybe somebody is trying to fool you.
JUSTIN CURRY: So Latief argues, maybe somebody is trying to fool you. It's interesting. One of the questions I have, actually, in regards to chapter 6 that I handed out to you, is I asked you guys to Google the Voynich manuscript. And the Voynich manuscript-- and that's pronounced voy-nich-- has been the subject of intense interest by cryptologists for a good 100 years or so. All the guys who were working during WWII to crack the German enigma code would in their free time try to decrypt the Voynich manuscript.
Because it was this very strange, medieval texts that had this really intricate system of writing. And it had then pictures of all these sort of alien plants and weird things like this that were not at all found anywhere in Europe, but the book was a European book. So people are wondering, what the hell does this mean?
And the problem really wasn't solved until just about a year ago when somebody showed how you could produce almost an exact replicate of the Voynich manuscript using a random generator. And you would just have a prefix for a word, like go, and then you would have them a mid-fix-- I don't know what let's call-- g-l, and then a suffix. And then you would create a matrix of these things, and you would shift around and just randomly start generating words. And then they would appear to look like natural intelligible words, but they actually meant nothing.
So the Voynich manuscript actually contained no real meaning, but it seemed to trick everybody because it had a lot of the same patterns that human language did. There's some other interesting things that's connected to parsing information. But I don't really have all the time to go into one of these things.
Speaking of w's and letters, there's actually something called Zipf's Law, which every kind of language or meaningful message appears to obey. And that's, if you rank the most common letters-- like, I believe e and some of the vowels are the most common letters-- that they follow a power law distribution, which means that the second most common letter is exactly half as apparent as the first one, and then so on. And it follows this very strict mathematical relationship.
And they've even run these kind of power law detections on DNA, and extracted that it appears to follow the same behavior as our natural languages do. And if you feel like googling that, that's Zipf's Law, Z-I-P-F.
But there's a lot of things going on here. And I want to return to the question, what does snow is white mean? And breaking down that question, what does snow mean? When I say snow, what do you think?
AUDIENCE: I think of snow.
JUSTIN CURRY: You think of snow. OK. But what does that mean? Let's avoid circularity.
AUDIENCE: Think of a picture of snow.
JUSTIN CURRY: OK. So Latief thinks of a picture of snow. What happens to Latief is a visual image is prompted in his head. Somewhere in his tangled brain here, the visual section of his brain is activated, and he has a visual memory of snow, or the first time that we had a large snow or a blizzard, and the first time you ever saw snow. What about anybody else? What does snow mean to you?
So then we've got in some ways a tactile sense of cold. So then another part of Latief's brain here lights up when you say snow. And it goes to all the times he's ever felt cold-- maybe just sitting in this room and having the air conditioning up a couple of notches too high. You have a feeling like, jeez, it's freezing in here. And freezing then triggers the idea of frozen water, which triggers the idea of snow.
But what else? What does snow mean to you? Some of you might see it as, oh, well, this is an opportunity to go snowboarding or something. Sorry, here's my bad recreation of bindings-- or go skiing or something, or it's recreation. It's time. It's winter. It's a break from school.
There's a whole really complex conceptual network in everyone's brain with the word snow. Just like if I were to pick apple, and I ask people, when I say apple, completely out of context, what is the first thing you think of?
AUDIENCE: Pie.
JUSTIN CURRY: Apple pie. OK. Anyone else?
AUDIENCE: Orchards
JUSTIN CURRY: Orchards. Anyone else?
[INTERPOSING VOICES]
JUSTIN CURRY: Red.
AUDIENCE: Adam and Eve.
JUSTIN CURRY: Adam and Eve. I'm glad someone said it, because I thought I was going to have to. So what then do you associate with the apple and Adam and Eve? Say it.
AUDIENCE: God.
JUSTIN CURRY: OK. So then we've got-- wow. Holy mackerel. We've already gone from apple to God pretty damn quickly. Maybe I should draw that off of Adam and Eve if we want to make it seem like that was the track we fell. But then what was it that Adam and Eve are being punished for feeding the apple? What was it the tree of? Knowledge.
How did Newton discover gravity?
AUDIENCE: Apple.
JUSTIN CURRY: What computer am I using?
AUDIENCE: Apple.
JUSTIN CURRY: A Mac. Excellent. Or your iPod, or whatever. So immediately, if somehow I could look at your brain, and kind of take an ongoing PET scan of what's lighting up as I say the word apple, it would be this extremely complicated explosion of electrical activity in your brain that lights up and tickles every part of the section of your brain that relates to the term apple.
Now, of course, when I have a frame of message, like this apple is tasty, then you immediately narrow it down. Well, what is he talking? He is thinking about just a fresh apple, a red apple, a Granny Smith apple? Is he talking about eating apple pie? So you prune kind of your ongoing neural net, and you stick to this side.
So I've noticed that nobody has come and claim their dollar or two. And this is, I think, a convenient point to pull out a dialogue we did a long time ago. Does anyone remember the contracostapunctus? And just as an apple has multiple meanings, this dialog, page 75, has a variety of meanings.
I need someone to start reading off the first letter of every sentence that Achilles, Tortoise, Achilles, Tortoise says.
AUDIENCE: H-Y-- [INAUDIBLE]
JUSTIN CURRY: Sorry, sorry. So I think it's H-O. Right, so we go--
AUDIENCE: Oh, oh right.
JUSTIN CURRY: Yeah. So go Achilles, Tortoise, Achilles, Tortoise.
AUDIENCE: H-O-F-S-T-A-D-T-E-R. S-C-O-N-T-R-A--
JUSTIN CURRY: On second. S-C-O-N--
AUDIENCE: S-C-O-R-A--
JUSTIN CURRY: S-C-O-- Sorry. S-C-O
AUDIENCE: S-C-O-R. Oh, I'm sorry. S-C-O-N-T-R.
JUSTIN CURRY: N-T-R.
AUDIENCE: A.
JUSTIN CURRY: I think I have too many S's here. A. C.
AUDIENCE: R.
JUSTIN CURRY: R.
AUDIENCE: S-T-I--
AUDIENCE: There's another--
JUSTIN CURRY: O-S-T-I--
AUDIENCE: P.
JUSTIN CURRY: P.
AUDIENCE: N.
JUSTIN CURRY: U-N.
AUDIENCE: C.
JUSTIN CURRY: C.
AUDIENCE: [INAUDIBLE]. A-C-R-O-S-- [INAUDIBLE].
JUSTIN CURRY: A-C-R. S-T-I-C.
AUDIENCE: A-L-L Y-B-A-C Y A-R-- [INAUDIBLE] S-S-P-E-L-L-S. J.S. B-A-C-H.
JUSTIN CURRY: Did anybody see this? So I should have asked it as a homework assignment, but one of the things that Tortoise and Achilles says is, starting on the page of 81, he says, there are many, many clever ways of hiding things in music-- or in poems. Poets used to do very similar things you know, though it's rather out of style these days. For instance, Lew Carroll often hid words and names in the first letters, or characters, of the successive lines and poems he wrote. Poems which conceal messages that way are called "Acrostics."
So we already had kind of a frame and outer message screaming out-- saying, I'm talking about me, sillies. Decode me! And already from the dialogue, which had it's one level of meaning, it then, within itself, pointed upwards to a higher level of meaning, which is this acrostic we just pulled out.
Well, what does the acrostic tell us to do?
AUDIENCE: [INAUDIBLE].
JUSTIN CURRY: Sure. And what does that get us? Sorry-- acrostically. There shouldn't be a space here, so join that over there. Let's see. Well, if we then look at this as a another acrostic, and we take the first letters of each word, what do we get?
AUDIENCE: JS Bach.
JUSTIN CURRY: And then the third, and I think probably the highest level meaning we can extract out of this, is JS Bach. So to what extent does contracostapunctus mean JS Bach? And there's, I think, a lot of really neat stuff going on when he kind of asks about messages which can talk about themselves and also build themselves. And that's basically what we did here, is we had a dialogue which gave us instructions on how to extract meaning from this. And then it in turn gave us another message which told us how to extract meaning from it.
And in some ways it's a really inefficient thing to do, because what was a 10-page dialogue, that much was needed in order to extract the inner message, or one of the inner messages here, being JS Bach. So that's kind of a poor compression ratio here. It took 10 pages to tell us one name. But I think it's interesting nonetheless.
But still, not everybody picked this up. So to what extent does this dialogue mean JS Bach? Well, I would argue not to a large extent. And fundamentally, what I'm going to argue here-- and this kind of goes with the Latief hypothesis or conjecture-- is that meaning is not inherent.
And to expand on this-- and this is what Hofstadter calls the jukebox theory of meaning-- meaning is the relationship of things. But what does that mean? What I'm trying to argue here is that if we wrote apple on a piece of paper, and we shot it out into outer space, and maybe some alien civilization came upon it, that there's not really much inherent meaning in having a few ink blotches on a piece of paper, or in here I have some limestone broken on to a piece of rock. To what extent does limestone broken onto a piece of rock mean anything?
And the only reason we say apple has meaning, is because there's this complex isomorphism between the visual input of apple when you read it to the electrical activity in your brain. Just like-- and I don't actually know any Chinese here-- I can try to construct a character, and to what extent does that mean something? And I could have someone sitting in here and say, well, no that's actually the Chinese symbol for tranquility. But how was I ever supposed to know that. How was I supposed to discern this from nonsense?
And really, this shows that meaning doesn't lie here or here, but it lies here and the relationship of things. So the extent to which this means anything is the extent to which it has a direct correlation in the minds of the people using the terms. It's just like when you have these native languages and they die out-- they no longer carry meaning, or really they continue to exist in any way, shape, or form, is that you don't have people actively using and kind of interplaying in this complex feedback process of modifying what these things me.
Also, think about how these symbols, how these marks on this limestone have changed meaning. If we were to rewind 100 years ago, apple would definitely not mean laptop. And I don't know if the myth involving Newton and his discovery of gravity-- which is a myth by the way-- existed 100 years ago. But let's suppose it did. But let's rewind 500 years ago. Then this would absolutely have no connection with the apple. And we would be the supported neural network of what this string meant.
So fundamentally I'm arguing here that this only means as much as the stuff it causes here. But this brings kind of a reverse question, with an isomorphism you go two directions. So suppose instead-- and this is really what a lot of neuroscience would love to do-- I just had a camera running of all the activity going on in your brain, and I wanted to reveal your thoughts. To what extent could I say, firing in sector b, a 6 1 5 G means apple? To what extent can I say that?
And this is actually going to bring forth a really important question, which will be the exploration of the rest of today's lecture. To what extent does physical activity have meaning? How do we distinguish between random physical things and pattern physical things? What was it when Galileo was bored in church and he looked at the pendulum rocking back and forth, and he would use his heartbeats to keep track of the period of the swings. And he noticed that it was always the same. And it didn't even have to do with how big this mass was here. But it seemed completely based on the length.
Now, In some ways, what is the meaning of a swinging pendulum? And if I wanted to describe a pendulum to you, I could do a variety of things. Just like I could ask, well, what does the firing in these in your brain mean? Ideally, in the future we'll be able to say, well that particular sequence of firings in your brain meant you were thinking about an apple. But what happened, fortunately, a couple hundred years ago, was that somebody was looking at this and then they realized that they could do better.
Instead of just filming the phenomenon, instead of just saying, OK, what happens when a pendulum swings. Well, the best level of description we can do is a streaming 256k video webcam of a pendulum-- that's a really bad compression to encode information. Ah, keyword-- information. Fundamentally, what I'm going to argue, we're pulling this down to, is to what extent is there information in here? And the study of theory of meaning and information theory is going to be our mathematical and rigorous approach to what the theory of meaning is.
So how much information is in this? And what it took was that somebody eventually realized, well, I can describe this complex motion actually rather simply. Well, if I looked at the angle theta that it makes, I could say-- and I apologize to you who haven't had calculus-- when I have a dot about the symbol, I want to see how quickly it changes with time.
So if this were just a single dot, I would mean what's the change in time of the angle theta. But if I had two dots, I'm asking, what's that change in time of the change in time of the angle theta. And you could think of this as acceleration. Whereas a single dot would correspond to velocity.
I can actually write down the equation of motion of a pendulum as such-- theta double dot plus, and this is just a constant, sine theta equals zero. And then if any of you, as you eventually will learn, is that this is too hard to solve as an equation, but instead we express it in a linear form-- and don't worry if you don't know t any of these terms mean-- where we approximate sine theta, which some of have seen precalculus, or even geometry, as just theta. Around zero we can approximate sine theta-- passing through the origin, sorry-- as just the linear function of theta.
But the point-- don't worry about the mathematics here, I could be talking any system and we'll show you today-- is that what the project of physics is, and what the project of all of science is, is it's reverse engineering. All of Hofstadter has been talking about in chapter 6 has been going one direction. And you start with a string, and you ask, well, what does it mean? And my answer was that what apple means, is it means the activity in your brain.
And then we can say that it actually refers to the physical apple somewhere out there in the universe. But that becomes a difficult problem. But then what about going the reverse way? To make extent does the activity in your brain mean this-- is the same question-- which Hofstadter doesn't talk about-- as, to what extent does the motion of a pendulum-- just kind of rocking back and forth. Let's see, do I have any-- here we go.
To what extent is the meaning of this motion encoded in these marks on a piece of paper? And this is actually, in some way, a much harder question, is how do we take the output and code it into the input? And what we're going to be doing today is playing with some active computer simulations and showing to what extent does the output we see on the screen, can we figure out just from that what was the underlying mechanism. And really, what all of science and physics has been about is showing that the complicated motions of the entire universe can be explained very shortly using symbols like this.
And this is going to get to an idea that we're going to call algorithmic complexity. What's beautiful about the pendulum is that it doesn't require a streaming feed all the time to describe its motion. I can write it down in one line of mathematics and give you the complete time evolution of the system. And even with the initial conditions of how fast was I swinging it initially from what place, I can get to this whirling phenomenon and get all sorts of incredibly complex behavior out of just these marks on a blackboard.
And then similarly, when some of you were here two lectures ago, and you looked at the Mandelbrot set, an extremely complex fractal, what if somebody had handed you that, and said, tell me what equation produces this. For the most part you couldn't have done it. What, Latief?
AUDIENCE: It seems like an equation is sort of hiding the information or something. And because you just look at the equation by the [INAUDIBLE] we talked about [INAUDIBLE] So you just [INAUDIBLE]
JUSTIN CURRY: OK. So Latief said, well, isn't all of mathematics just really hiding the information. And what I'm going to kind of do, and correct me if my interpretation of what you said is wrong, is that really all we're doing is we're taking our mental process-- our visual input-- and we're abstracting away the details. We're creating an abstraction barrier between that and this.
But I would argue to an extent, no. Although fundamentally this sentence is only meaningful to mathematicians and thus the models they have in their brain, but this actually reveals a very simple relationship. So what happens when we talk sine theta, how would you parse that? Well, you might draw us a circle, and then say, OK, if I had a triangle here, I would say that sine theta is opposite over the hypotenuse. And the fact that this has a relationship with that involves some serious thinking.
But yes, you are masking some of the details. But if you wanted this to run on a computer, it would be much more efficient to just code this up in a programming language than to take a film.
AUDIENCE: So you can do that. But wouldn't you [INAUDIBLE] find out what those symbols actually stand for, it turns out that you're not really abstracting it too much. You're just making these symbol just stand for things.
JUSTIN CURRY: OK. So Latief's saying that, fundamentally still, what we're doing is we're letting the symbols stand for the thing itself. But I'm still going to argue against you. And the reason for that is that there is something unifying behind looking at the arm swinging and a clock and what I'm doing with this cable. And then as you'll start to study more-- and as we'll show today-- throwing a rock in a puddle, and then just getting this entire wide class of oscillatory phenomenon. What is it like to do this?
And we're going to see it everywhere. And you can really observe this when you're driving. And then we going to get into wave equations. The idea of when you're driving down a highway and you hit a backed up section of traffic, what's happening is that the guys in the front stopped, and that forced you to stop, and that force the car behind you to stop, and you propagated a wave backwards.
But still, we can describe this entire large set of seemingly unrelated physical phenomenon. We're not talking about this single clock. We're not talking about the single pendulum. We're not talking about this single table. We're talking about a whole variety of phenomenon and just writing down this equation.
And I think that's a really neat thing. But this is a phenomenally hard thing to do. It's hard to see all of these classes of phenomenon. It's hard to see me throw this piece of chalk in the air and extract the equation which describes it. So what we're to spend the rest of today talking about is not how do we go from apple, or how do we go from the equation, to figuring out, well, what does it mean.
And it's the same question of does the equation y equals x squared really mean the graph of x squared? And you're going to see that, no, it doesn't always. It can mean a whole variety of things. I don't even have to graph it. I could be talking about a different domain and all sorts of different phenomenon. But it's kind of the relationship of these different ideas that we're going to be talking about.
And we've got some great things to show for you. And for the most part, I'm going to be handing this-- sorry. Yes, Latief.
AUDIENCE: Isn't it, when you see all of these similarities between different structures, aren't we making stuff up [INAUDIBLE]?
JUSTIN CURRY: So are we making stuff up. Yes, we are making stuff up. Because we're only modeling things here, right? If you actually then measured does the rate of change of the rate of change of the angle-- this cable-- always equal minus sine of the angle? And for this system, obviously, no. Because look, it's bending. And it's got all sorts of weird, elastic properties. The rod's not stiff, it's actually flexing about and doing all sorts of crazy things.
And this isn't a massless rod here with just a big ball on the end. This thing has got almost as much mass as the end. So, no. But the fact that we can model most of the behavior, most of the salient features of the phenomenon, in a rather simple way is one of the remarkable things about science and about mathematics.
So just to kind of convince you that complexity can come out of simplicity, you might say, OK, well, doing this isn't really that complex. I mean, I probably could have figured out a simple way to describe it-- go ahead.
AUDIENCE: So that shows something about the universe over [INAUDIBLE]. Because, you see, maybe the universe is [INAUDIBLE].
JUSTIN CURRY: OK. So Latief says, maybe the universe is doing something different, and we're just showing how it can be done simpler. That's a good question. But the problem is is that the most we can do-- the way that we fundamentally think as humans, since we are such simple creatures are we're not the universe-- well, we're part of it-- is that we have to be able to simplify our phenomenon in ways like this. And if you ever end up reading a book by Seth Lloyd called Programming the Universe, you'll say that the universe itself is a quantum computer carrying out quantum operations all the time.
And maybe somewhere in there, at the beginning of the universe, there was a monkey typing away instructions into the quantum computer that is the universe, that said, OK, well, we're going to let force always equal mass times acceleration for those we have done physics. And we're also going to let this, and this, and we're going to-- and this was all hard coded in there. But the issue is, we don't know that. We don't know what happened exactly at the beginning of the universe.
We don't know how exactly the universe operates. There are still mysteries out there. And the fact that we can go from throwing this piece of chalk to understanding what's the law which governs that, is really a remarkable achievement of humankind.
But I want to really hammer home this idea that complex phenomenon have a very simple underlying feature. And this is going to relate to some different ideas of information theory, which I might periodically pop in and invade on Curran's time here. But for the most part, he's going to take over the show now. But should we take a quick break?
CURRAN KELLEHER: Yeah, let's take a break.
JUSTIN CURRY: All right. So let's come back in probably five minutes and refresh ourselves. And then Curran will take over.
CURRAN KELLEHER: OK. So I'm going to start talking. So this is the Sierpinski triangle, which I talked about last time. And there are a lot of different ways to get to this shape. So he's talking about, how do you go from seeing this shape to understanding the process of how to do it. It's not an easy thing.
This is what's sort of unique to humans, we can step back and say, OK, what's going on here? What kind of more generalized process might lead to this thing? So I'm going to talk about a bunch of different systems-- Lindenmayer systems and cellular automata, and a bunch of examples of each.
So first of all, I'll just do an example of a Lindenmayer system. A Lindenmayer system is when you start with a string. A string is just a list of symbols, like characters. And you apply these rewrite rules, and you have this grammar. So each one of the symbols goes to some other set of symbols when you rewrite it. So I'll just do an example.
We start with F, and we have these rules. F goes to-- this means it's a string when put in quotes. "F" goes to. So whenever we have this symbol "F," we replace it with "F plus F minus minus F plus." And does this mean anything to you guys? Probably not. So I'll just go through it.
So "F," when we apply the rule for first time, what we get is this-- we get "F plus F minus minus F plus." So here's when we really get to the recursive nature of Lindenmayer systems. We feed this string back into the rule. And so for each F here, we replace it with that.
So this F, the first F, becomes F plus F minus minus F plus. So that's what this first F becomes. Then we add plus the second F. And we do that whole string of stuff again, minus, and then another repetition of that, and then plus. And we just keep doing this.
And now let's recall the Koch curve from last time. What we do is, first thing, we have this-- just a line. And we apply this rule that says we make this little notch in it. So we get to this. And then, we apply that same rule to each one of these things.
So what does this mean? Does anybody have any idea what this means? These symbols? So the meaning comes out of the isomorphism, like he said. How do you interpret them?
So F, in this case, stands for going forward. What?
AUDIENCE: [INAUDIBLE]?
CURRAN KELLEHER: Yeah. This and this are actually the same thing. This is the power of Lindenmayer systems. So F stands for going forward. And plus stands for changing the angle by 60 degrees. And minus stands for a changing the angle by minus 60 degrees.
So first we do-- the first F is just this. This is F. And this is the second one. So F, forward plus 60 degrees. F plus 60 degrees, F minus 60 degrees, minus 60 degrees again, F plus 60 degrees F. Sorry, there should be an F on the end. This is what you get.
The way this is taught, is think of a turtle. And a turtle has an x, y, and a theta-- a position and a rotation. So each symbol is interpreted as having some sort of effect on this turtle. So I wrote a program that does exactly this. So let's look at that-- the Koch Lindenmayer system.
So I'll just quickly go over the code. So we have turtle x, turtle y, turtle theta. This is the important thing. This is where the turtle starts.
So we start with this string F. And so this is F, the first string. And for int i, i less than depth i equals plus. This just means do this certain thing depth times. And depth is a variable.
So when I click on the screen, depth increases by one. String, which is F, equals string-- what the string is now-- replace everything in that string by this specific thing. Replace F by F plus F minus minus F plus F. And then, we interpret it-- we give the symbols meaning by this isomorphism, how we interpret it.
So for each character in the string, if the character is F, then what we do is we store the previous values and we move forward. And cosine of sine it's just how we interpret the angle-- go forward times the increment, which is the length that we go forward by. And we add a new line with those x and y values.
So this is what F means. And then plus, what it means is just turn the turtle a little bit-- turn the turtle's angle by pi over 3. And likewise with minus, subtract from the turtle's angle, pi over 3. So we can run this and see what it looks like.
And I added some more things like mouse listening. So first of all, it's printing out the strings. So this, right here, if you can see it, says F. So I'm going to click, and it does that-- just the rule that we were talking about. And now, the string says F plus F minus minus F plus F.
And when it's interpreted, it gives you this picture. So click it again, apply the rule, feed; it through-- we get this. And it prints the string here. So this is the next string. And then we do it again, and the string gets longer.
So we can do it again and again and again, and we get the Koch curve, which is this strange curve that has infinite length. Which is pretty wild. Any questions so far?
So a Lindenmayer system is very general. Yeah?
AUDIENCE: Can you go back to the picture?
CURRAN KELLEHER: Can we do the picture again? Sure.
AUDIENCE: What if you see [INAUDIBLE]? Is it going to be the same?
CURRAN KELLEHER: What, what?
AUDIENCE: Like, flip the picture over and see [INAUDIBLE].
CURRAN KELLEHER: If you flip the picture over?
AUDIENCE: You have a certain shape, what if you take the other shape? If you have no other boundaries, [INAUDIBLE]
CURRAN KELLEHER: You come up and motion, and show what you mean? If you were to view this curve as starting here and going to here?
AUDIENCE: Yeah. Would it be the same?
CURRAN KELLEHER: And it's upside down? It's the exact same thing. Yeah. That's why it's a fractal. There are copies of itself inside of itself, nested infinitely. That's what it means to be fractal.
So for example, this whole thing is this right here. This thing is the same as the whole thing. And likewise, a smaller version of what you were saying. But if we flip this upside down to here, it's the same thing. Yeah. So, yes. Isn't that cool?
So here's another Lindenmayer system which is a bit more confusing, I think. But it's the same idea. It's a rewrite system-- Lindenmayer system. So we start with the string A, and then the rule for grammar is, A becomes B minus A plus B, and B becomes A plus B minus A. And the string becomes that.
And what these things mean is if it's A or B, go forward. And plus, turn the turtle one way, and minus, turn the turtle the other way. And so what we get here-- I'll run this, and we can see what it looks like.
So first we start with this-- just a line. And we apply the iteration. This is twice actually. Because if you iterate it once, it goes like upside down and off the screen, so I just did do it. So this is after two iterations.
So think carefully about the shape. So I'll just iterate it again. And we'll notice that from each one of these lines, this shape is going to grow again, only in alternating directions. So the original line was here, here, here. So here it grows this way, here it grows that way, here it goes that way-- it goes that way, and there it goes up.
AUDIENCE: It's like it's interlocked within itself.
CURRAN KELLEHER: Yeah. It is like it's interlocked with itself. So I'll iterate it once more. And from each one of these little lines is going to stem one of those shapes again. And as it's approaching the Sierpinski triangle. So we'll do it again-- do it again. There. So it's just a bunch of little squiggly lines, but it's becoming the Sierpinski triangle. So, yeah?
AUDIENCE: Did you say F string, or what string would you use?
CURRAN KELLEHER: For this?
AUDIENCE: Yeah.
CURRAN KELLEHER: So this string is not F, but it begins with A. It starts with A here, and it applies these rules to it. So this is the printout of what the string is. So first it's A. After two iterations it becomes this. And then every A in the second string-- every A here-- is going to be replaced by this thing. And that's what we get with this one, it just goes off. But it's a similar concept. So this is the string.
And likewise, with the tree, I had a tree example last time. And we can make a Lindenmayer system for a tree. And the string is FBR. So if we look right here, the string is FBR.
And the rule is, every B becomes this. So we can really think about this and give these things meaning by interpreting them. So F means go forward, B means there's a bud on the end of the branch, and R means reverse.
So let's think of building a tree. We're going forward. So F, and on the top there's a B-- bud-- and then R-- reverse. And the rule is, each B becomes this. And we'll see what this is-- what this means. Each B becomes minus FBR plus plus FBR minus. So each B, each bud, becomes minus FBR plus plus FBR minus.
So let's run this and see how it looks. Anybody have questions so far? Yeah.
AUDIENCE: So why are all your strings palindromes.
CURRAN KELLEHER: Why are all the strings palindromes? Wow. They're palindromes, because each side of the tree is exactly the same. That's why. And by the way, if you don't know what a palindrome is, it means it's the same forward and backwards. Is that what it is? Right? And it makes sense, because the tree is the same if you flip it around.
So I'll run it. So the first thing is FBR-- forward, bud, reverse. Do it again, and it's this tree. There we have it.
So look at this picture. And I'm going to show you this other program, that I explained in my last lecture, with this recursive function that says tree and grow tree with a smaller size. And I'll run this. And lo and behold, what we get is the same.
This is the Lindenmayer system. And this is the recursive function. They're exactly the same. So what does this mean? There's something deeper about fractals? You can approach them from different angles and get the same thing. I'm just fascinated by it.
So I'm going to move on to cellular automata now. Any questions on Lindenmayer systems? So cellular auto-- yeah?
AUDIENCE: [INAUDIBLE] you get the same thing? Does Lindenmayer system equal-- do they have an isomorphism relationship with fractals.
CURRAN KELLEHER: Yeah. So your question is--
AUDIENCE: If they're approached in different way and they equal the same thing, do those two different approaches equal each other.
CURRAN KELLEHER: In some way, yes. So what you asked is, so you have two different approaches, and if they approach the same thing, are they in fact the same thing? More or less is your question?
AUDIENCE: Yeah.
JUSTIN CURRY: So it's the idea that, really, we have two different descriptions for the same phenomenon. So to what extent are those descriptions the same?
CURRAN KELLEHER: So, right. I mean, it's sort of mind boggling. We have two different descriptions for the same thing. So to what extent are the descriptions the same? They are definitely different, but they describe the same thing. So the thing that they're describing is the same, but they themselves are different. It's hard to really say. Yeah?
AUDIENCE: It seems like this sort of describing an [INAUDIBLE] object in visual terms [INAUDIBLE] terms?
CURRAN KELLEHER: Say it again.
AUDIENCE: Like, describing something as visual and then [INAUDIBLE] audio [INAUDIBLE]
CURRAN KELLEHER: Describing--
AUDIENCE: [INAUDIBLE]
CURRAN KELLEHER: Ah, OK.
AUDIENCE: Or something like that.
CURRAN KELLEHER: So he's sort of making the analogy to describing something in reality using words, or using pictures, or using sound, or using text. So yeah, what you're getting at in all these different descriptions is the same fundamental thing. This is the inner message that Justin was talking about earlier. You have the frame message, the message, and the inner message. The message is the real meaning-- the thing itself.
AUDIENCE: Could also the physical thing be like another way of describing something more abstract? Like this [INAUDIBLE] describing the [INAUDIBLE]?
CURRAN KELLEHER: Oh, man. So he said, so maybe physical reality itself is just a description of something else-- something else more--
AUDIENCE: Like, mathematics is also another description--
CURRAN KELLEHER: Mathematics is also another description of--
AUDIENCE: Maybe mathematics itself is the thing that's being described.
CURRAN KELLEHER: Maybe mathematics itself is the thing being described by the physical universe. I mean--
JUSTIN CURRY: Looks like we have a Platonist on our hands.
CURRAN KELLEHER: We have a Platonist on our hands.
[LAUGHTER]
Yeah. So it's sort of like asking the question-- so all of these different descriptions of fractals all lead to fractals, what does it mean? Is the fractal itself describing something deeper? I mean, I don't know. We sort of get that sense, but it's so elusive. Yeah?
AUDIENCE: Dig deeper into the fractals, since it's recursive itself? [INAUDIBLE]
CURRAN KELLEHER: Can you go deeper into the fractal?
AUDIENCE: Like, a definite--
JUSTIN CURRY: It's scale-free. Yeah. It's scale-free.
AUDIENCE: The fractal itself is--
JUSTIN CURRY: Contained inside.
AUDIENCE: Yeah.
CURRAN KELLEHER: Yeah. Yeah, you can go out into the fractal infinitely. But what is it-- what are the implications of that? I don't know.
AUDIENCE: Where does it lead to.
CURRAN KELLEHER: Yeah, where does it lead to? It leads to more of itself, I guess. It's recursive. You know, there's no end. Yeah?
AUDIENCE: If the universe can almost affecting in a [INAUDIBLE] itself, it has [INAUDIBLE] And at other times, that's [INAUDIBLE]
CURRAN KELLEHER: Could the universe be a fractal in a more abstract sense? Yes.
[LAUGHTER]
AUDIENCE: [INAUDIBLE].
JUSTIN CURRY: Yeah. I just have to butt in there. Because before we had kind of a quantum mechanical version of the atom, what we used to say is, here's the nucleus, and here are the electrons. And, of course, I could have said, instead of nucleus, here's the sun, and here are the planets. So in this sense-- now, once again, this model isn't right, but what we used to have is this kind of self-similarity across scales.
If we zoomed in far enough from our solar system and went from a solar system and just kind of getting telescoping in and in and in and in, visually, until we got to length scale on the level of atoms, I could replace this and it would look exactly the same. And I would say, this is the nucleus, and here are the electrons.
Of course, this isn't exactly right, but what's interesting is that a lot of physical phenomenon-- and this is a very kind of a deep experiment, is that-- and I know Curran talked about this a little bit-- if you take a mountain and you look at the shape of a mountain as kind of this crinkled, fractally thing, and then you take a piece of paper, and crumble it up, and then unfold it, the geomorphology of a paper is almost exactly the same as a mountain and the landscape around it.
So in some sense, there's a self-similarity in conceptual laws between the forces that govern the shaping of mountains, valleys, and rivers, and the forces behind me crumbling this piece of paper.
CURRAN KELLEHER: And also, it's so inspiring in biology, you see these patterns at different levels on different scales. And they're all the same. They all share something. So biology, like yourself-- like your own body-- is definitely a fractal in some sense. Not infinitely, but there's all these-- yeah?
AUDIENCE: Could it be like the thing itself, or the way we describe the thing.
JUSTIN CURRY: Ooh. So Latief said, could it be the thing itself or the way that we describe the thing?
AUDIENCE: Yeah.
JUSTIN CURRY: So then that fundamentally boils down into, which do you think is more real, the pendulum or the equation describing the pendulum?
AUDIENCE: It's the chicken and the egg example, right?
JUSTIN CURRY: So Sandra says it's the chicken and the egg example.
CURRAN KELLEHER: So speaking of biology and life, life is complicated, right? Life is complex. Societies just evolve, and people do things, and you can't really predict what's going to happen. And you have all this complex behavior. But in society it sort of arises from complex rules of interaction between people.
But with computers and cellular automata particularly, what we can do is very, very loosely model life. And we get this. And it's similar to actual society in that it's very complex behavior, and we can't tell by looking at how it's going to end, and what's going to end up. Maybe small things could balloon into huge influential events. Like, one person influences the whole future. It's fascinating.
So what I'm going to do is talk about these rules, and what this program is, and how it works. So this is Conway's Game of Life. It's really cool. So we have this grid of boxes, and each box is termed a cell-- cellular automata. And that's automata because it evolves-- it keeps going, based on simple rules, autonomously. It does it by itself.
So you have this huge grid. And you can think of each cell here as though it were its own little organism just sort of going through life. So let's consider this one. If we are this cell-- a cell is a place for an organism to exist or not exist. So there are some very simple rules.
JUSTIN CURRY: I have them written down.
CURRAN KELLEHER: You have them written down? It would be good to just have a piece of paper. So a cell is either filled in or it's not filled in. Say we are this cell right here. If we're alive and there's only one cell around us, we die of loneliness. And so, this cell would die.
If there are two cells, it's OK. It's a healthy sort of environment. Like, those are our parents maybe-- and we live. We're this cell. If there are three-- maybe it's our parents and a brother or something-- we live. But if there are four-- and these numbers I'm talking about are the total number of living cells in our neighborhood. So these surrounding eight cells is the neighborhood of this particular cell. So if there are four living cells in the neighborhood of this one, then it dies of overpopulation-- suffocation. Four or more. If there are more then the cell dies.
And if the cell what?
JUSTIN CURRY: Yeah, good.
CURRAN KELLEHER: If the cell is not alive, then if there are three in its neighborhood, then it comes alive. So if there are three cells around it that are alive, then they birth a child or something. So this is the entire rule set. These very few number of rules that makes this thing happen.
So it starts off with just randomly filled in cells. Oh, no, what have I done? It starts off with just randomly filled in cells. And let's just look at the code quickly. Cellular automata-- so neighboring cells is getCell x, y plus 1. And we go through this list of things. We basically get all of the cells in our surrounding neighborhood and put them into a list.
This notation means array. This is like a list of cells. A cell is an object which I made, which has a property of either being alive or dead, basically. And we're drawing it as a square. And so, if my value is 1-- my, being the cell that we're considering at the moment. And mind you, this is inside of a loop. Note this double double for loop, for each x and for each y. So the stuff inside of these double for loops is going to be executed once for every cell.
So let's look on the inside. So my is the cell at x, y. If my.value is 1, meaning that I'm [INAUDIBLE] I'm alive-- there's something living in this cell. If they are less than two, then I die of loneliness. If there are more than three, then die of overpopulation or suffocation.
So this can be encoded is if this neighborhoodSum, which we calculated a few lines ago-- just added up for each cell in neighboring cells-- neighborhoodSum, is you add that cell's value. So for example, with this cell, the neighborhoodSum would be 3-- the number of living cells around it. If my value is 1, then if the neighborhoodSum is 2 or 3-- so if there are two things around me or three-- then my nextValue, the value that I will be in the future, is going to be 1. I'm going to stay alive. So otherwise, I'm going to die, my nextValue is zero.
So else if my.value is 0-- this means if the cell is originally dead, if the cells is originally black, there's nothing there. In that case then, if the neighborhoodSum is exactly 3, then my nextValue is 1. Otherwise, I stay dead. So if there are three people around me, then I'm going to be born into this cell.
That's basically it. And we calculate the colors and whatnot. But that's the essential piece of the program.
And when we run it, this is what we get-- Conway's Game of Life. Any questions?
AUDIENCE: Does it ever repeat itself?
CURRAN KELLEHER: Does it ever repeat itself? Well, we'll see now it's repeating itself every two frame.
JUSTIN CURRY: So it's actually hit a steady state now.
AUDIENCE: So it can go on forever-- it will never change.
CURRAN KELLEHER: At this point, yeah. It's in a periodic cycle, so it's never going to change.
AUDIENCE: Like if you put in a random guy to make it change.
CURRAN KELLEHER: So let's see, does my mouse click work? I don't know. No, it doesn't work. But it depends on the initial conditions, right? Let's run it again.
If we run it again, it might never do that, because the initial conditions are random. Think of this as a computer program. This is the halting problem of computer science-- Alan Turing's halting problem. So that basically said that-- and this is in the hand I believe. Is it Justin? Maybe not. I'm sorry.
But Alan Turing's halting problem says, if you have a given computer program, there is no computer program that you can write that will analyze that program and tell you whether or not it will stop. So stopping, halting, coming to an end, or coming to a stable point, is unpredictable. It's impossible to predict given a set of inputs whether or not the system will ever come to a stable point.
So this-- the configuration of cells initially-- is actually a computer program that's going to be set in motion when we start the simulation and start applying these rules.
AUDIENCE: So it can get the whole Turing machine thing just by moving this one [INAUDIBLE]. They're almost doing the same thing.
CURRAN KELLEHER: Say again.
AUDIENCE: A Turing machine-- [INAUDIBLE]
CURRAN KELLEHER: A Turing machine-- right. So, yes. Applying these rules can be reduced to a Turing machine. Yeah.
AUDIENCE: And a Turing machine can also be reduced to that.
CURRAN KELLEHER: What? A Turing machine is like the lowest level of existence of a computer program.
JUSTIN CURRY: Yeah. But I think it's equivalent. I'm not sure if-- I think Game of Life, but I know some other cellular automata rules are universal Turing machines So you can either think of it as a strip of paper which is just doing very simple computations back and forth or you could also do computation on the level above of squares and cellular automaton like this. And what that means to be a universal Turing machine is that you can fundamentally reproduce the basic logical operations of and, not, and copy. And from that you get, essentially, all of mathematics and all of anything you want.
AUDIENCE: [INAUDIBLE]
JUSTIN CURRY: OK. So (LAUGHS) that's kind of-- the touchy issue there is, can you derive all true statements recursively from a set of axioms? No. Godel's Incompleteness Theorem tells us this. And that's why mathematicians will never go out of a job, right? Fundamentally, there's always new truths out there that aren't reachable from your set of axioms. So you kind of have to go out there and meta think, and then discover it from a higher level outside of just recursive operations acting on axioms.
But interestingly enough, Godel's Incompleteness Theorem and the halting problem are really, fundamentally, kind of the same thing. Because the way you prove the halting problem is you feed the program which is supposed to decide whether or not it's going to stop, to itself. And it's that very recursive nature, and it's fascinating. We'll talk about it more.
CURRAN KELLEHER: So keep in mind that in two lectures from now, I think, we're going to really teach you what Godel's incompleteness is. So just wait for it-- it's coming. So I'm going to show you two more examples of cellular automata, and then I'll be done.
One of them is voting patterns. Voting rules is what it's called. And what it is, basically, think of people who talk to their neighbors and their opinion about which political party is the best is slowly influenced over time by those people around him. So think of, these are like shades of gray. This person is sort of in the middle. This person is really Republican or something. And then this person is really democratic. Let's just consider this.
So what the rule is for this-- in the Game of Life, it was binary. That means, there were only two states on or off, alive or dead. But in this situation, there are infinite number of states. It's a real number between negative 1 and 1.
So what happens is, this person has a number-- say, it's 0.5. And every iteration, he takes stock of the people around him and adds to his number the sum of his surroundings multiplied by some small number. So he's only influenced a little bit, but he's influenced by his surroundings.
And so, what we get is called coarsening. It's a coarsening effect. So the simulation that I'm about to show you starts off with complete noise-- it's completely random. Each cell is assigned a value between negative 1 and 1 randomly. And then we're going to apply this rule, and just watch it. It's going to be beautiful.
Isn't that awesome? So it's going to keep evolving. And the end state will either be all black, all white, or half black and half white divided somewhere. And it's just this very simple rule of summing the cells around you and adding to your state a very low weighted portion of their opinion. So we get this complex behavior. Who would have thought, right? That's really cool.
So I'll just go through the code quickly about this one. The structure is pretty much the same as the other ones. In these doubly nested for loops going through each individual cell, my is the cell we're at-- neighboring cells. Blah, blah, blah.
So this time, the cell's value is not 0, 1, but it's a real number. So we sum the neighborhood. And this line sums up the rule right here-- all of it. So it's my.nextValue is my.Value-- my current value-- plus the neighborhoodSum-- opinion of the neighborhood-- times 0.005. Yeah?
AUDIENCE: If you multiply by a lower number, [INAUDIBLE] it's just going to take less time to get to the same state.
CURRAN KELLEHER: Yes. So we can actually-- this is the beauty of having a live program. So this number, 0.005, determines the speed at which this system evolves. So if we make it 0.05 and run it, you'll notice that it will go a lot faster. It goes really fast.
AUDIENCE: It's the same thing, just twice as fast.
CURRAN KELLEHER: It's the same thing, just, actually, 10 times as fast, because it was 0.05 instead of 0.005. So we can go 10 times slower if we make it 0.0005. Let's make it 0.001, and it's going to go really slow. But that's the essence of the system, just be influenced a little bit by our neighbor. So it's just going really slow now, but it is still going.
And an interesting fact, cellular automata, similar to this one, are used a lot to do image processing and graphics. Like blurring, for example, is a cellular automata applied to the pixels of your picture.
JUSTIN CURRY: And I'd like to hop in there if you don't mind, Curran?
CURRAN KELLEHER: Yeah, go ahead.
JUSTIN CURRY: But this relates to a project that Curran and I did with the New England Complex Systems Institute. And this is kind of an example of what we call the universality class of phenomena. But the interesting thing is that this same rule-- this same behavior-- is actually what governs gas droplet condensation.
So if you look at the window of your car, and initially you have mist misting down on the surface of your window. What each water particle does is because it wants fundamentally lower its energy to want to take the path of least action. And based on surface tension, and the way that, essentially, the interaction between these water molecules works, is that water molecules like to be next to each other. Because it takes less energy to group together than it does for a bunch of water molecules to exist by themselves.
And I think it's interesting that the same rules and behavior, which simple things like water droplets on a sheet of glass behave, it's the same way that people behave. Fundamentally, it takes more work if you're just a hunter-gatherer by yourself, then to come together in society and grow collective farms, and then have someone responsible for this, and responsible for that.
So in this sense, we really do have universality class of phenomena. The same laws which govern-- and notice this is pure physics when it's in terms of water molecules. I mean, I can actually work out energy values. Yet, when we're talking about human society, you don't really have equations. But with cellular automaton we do. We have equations of how societies interact.
And one of the interesting things which the New England Complex Systems Institute is working on, is prediction of ethnic conflicts and violence. And you can use very simple models like this to predict where, if you're talking Gaza Strip-- you've got Palestinians and Israelis, how they mix and form together and then segregate each other, and what happens there. And this can all be described using this same kind of very simple graphical rules.
AUDIENCE: So we see complex [INAUDIBLE] or we see [INAUDIBLE] who don't understand the basic laws of the system, because it's always that it's almost the same [INAUDIBLE].
JUSTIN CURRY: OK. So you're asking is it just that we don't see the underlying laws behind society, and behind water molecules, and things like that? And that really is kind of an idea of which Stephen Wolfram, in his book A New Kind of Science voices, is that universe really is just this giant cellular automaton. And it's just cranking out these rules, very similar to The Game of Life.
CURRAN KELLEHER: Yeah. And I can segue very nicely from that into the next thing, which is waves. Now, cellular automata can do waves. And keep in mind that quantum mechanics is basically wave mechanics. Isn't that right, Justin?
JUSTIN CURRY: In a lot of ways, yeah.
CURRAN KELLEHER: More or less. And the interaction of particles can sort of be reduced to waves-- standing waves, in a sense. So let's take a look at this program. And imagine monkeys typing on a keyboard. What's the probability that a monkey will type this exact configuration of symbols?
AUDIENCE: Not very likely.
CURRAN KELLEHER: But it's a lot more likely than a monkey writing Shakespeare or something. Let's look at-- is this the right one? Let's look at this rule. This is really all that we need, this rule right here.
So let's look at the rule for us. And I'm going to ask you guys to try to predict what's going to happen. So in this situation, each cell has a height, but it also has a velocity-- a speed. So you think of it in 3D-- the height is the direction this way or that way, and the velocity is the speed at which it's traveling.
So looking at this rule, and the neighborhoodSum is the sum of the heights minus my.height. So the neighborhoodSum is the sum of the differences between my.Height and the cells around me. So for each cell in the neighborhood, the neighborhoodSum plus equals-- so that means add this to the neighborhoodSum-- the cell height for each cell around me minus my.height.
So if we're the same height, then it's going to be 0. If all the cells are the same height, then it's going to be 0. But if there's a difference, it's going to be the sum of the differences. And then my.velocity is increased by the neighborhoodSum divided by 8. So the average of the neighborhood.
So what does this mean? What is this going to do? Can anybody tell me?
AUDIENCE: If everybody [? launches ?] [INAUDIBLE] varied, you may have [INAUDIBLE] very low velocity.
CURRAN KELLEHER: Yeah, yeah. So if you're very different than the people around you, then your philosophy is going to be higher. So let's run it and see what happens.
So it's a wave, right? It's water. So it's fundamentally a wave. And since all particles are waves, it's something to consider that the universe may just be a giant computer. So I added mouse clicks to this, so we actually click on it. So if I click on the side, I believe it will work.
What's going on? Here we go. Look in the middle-- you have things going on and off, on and off, on and off. Hmm?
AUDIENCE: It's like a checkerboard.
CURRAN KELLEHER: Yeah, it's like a checkerboard sort of.
AUDIENCE: So we don't need all that calculus anymore.
CURRAN KELLEHER: Well, actually, what calculus is doing, is approximating exactly this. Or the other way around-- this is approximating the calculus. Calculus--
AUDIENCE: That's even more detailed [INAUDIBLE]. It's got more pixels in there.
CURRAN KELLEHER: Right. So he's saying, if you have more pixels and more resolution between the possible numbers that you can have, then you're going to approach actual physical reality. Yeah, you're right. But this is the fundamental limitation of computers that are discrete. So there's only a finite number of possible heights for each cell, and there's only a finite number of possible cells that we can have.
AUDIENCE: But the universe itself is discrete in a way.
CURRAN KELLEHER: How is the universe itself discrete?
AUDIENCE: I mean, time is discrete, space is discrete. And you have [INAUDIBLE]. It has to be discrete. It can't be continuous, because that means within [INAUDIBLE]
CURRAN KELLEHER: Well, the way I understand it, is the universe is continuous. Because take into account, I think, Zeno's paradox, right?
AUDIENCE: Yeah, but Zeno's paradox doesn't make sense. It only make sense when you [INAUDIBLE] and so, OK, when I walk from here or there, I'm heading up over small valleys or these different things that I have in a certain amount of time. So I am moving, because it's not [INAUDIBLE], it's discrete.
CURRAN KELLEHER: Well, what Zeno said is, to get from here to here, I have to first go halfway, and then halfway, halfway.
AUDIENCE: But it bottoms out to a single value.
CURRAN KELLEHER: But it does not bottom out. It goes on in infinitely.
AUDIENCE: Yeah, but that's on a line. We don't have lines in real life.
CURRAN KELLEHER: Well see, from here to there, it's a line. And--
JUSTIN CURRY: So fundamentally, the idea is, can we continue dividing matter infinitely small.
AUDIENCE: Maybe you can [? stop ?] a [INAUDIBLE]
JUSTIN CURRY: Right. I mean, that's a question, are strings the bottom level? We're talking in terms of string theory.
CURRAN KELLEHER: But what about space though? I mean--
AUDIENCE: Space itself,
[INTERPOSING VOICES]
AUDIENCE: [INAUDIBLE]. I mean, space is like [INAUDIBLE]. We don't have that capability. [INAUDIBLE] all the dots in there [INAUDIBLE].
CURRAN KELLEHER: Yeah.
AUDIENCE: You don't see that. It's just a way to show [INAUDIBLE]. You can still get [INAUDIBLE] this spot over here to that spot, you have to go this much distance. But that distance in the middle is like adding up like little, little distances.
CURRAN KELLEHER: Yeah.
AUDIENCE: But you can't get lower than those distance. Those are like the fundamental distances. That's why it's [INAUDIBLE]
CURRAN KELLEHER: So you're saying there's a fundamental smallest distance possible.
AUDIENCE: Yes. Otherwise, we wouldn't have [INAUDIBLE]
CURRAN KELLEHER: Right.
AUDIENCE: [INAUDIBLE].
JUSTIN CURRY: But then, here's the question-- in our Euclidean plane which is the universe, and this dot which is an atom, or something, or a particle, when it goes from here to here, does it ever not go through any plane in the middle?
CURRAN KELLEHER: There are an infinite number of points that it goes through. The thing itself may not ever be smaller than a certain distance. But see what we're modleing here is space, not matter. Here, space is defined in terms of these cells-- these discrete quantities. But in reality, there's an infinite number of little places at which the particle can be. So space is the thing that presents us with this huge divide between simulated things and real things.
AUDIENCE: So it's basically continuous, but it's [INAUDIBLE] is discrete.
JUSTIN CURRY: Potentially.
CURRAN KELLEHER: Potentially.
JUSTIN CURRY: Potentially, space could also be discrete. And that's kind of the computational view of the universe. Which, in some ways, you're advocating.
CURRAN KELLEHER: So yeah, if it's so small that we could never detect it, then the universe could be discrete, right?
AUDIENCE: Well, maybe space and time [INAUDIBLE]. Because in quantum mechanics, something [INAUDIBLE]. space and time.
CURRAN KELLEHER: So maybe more fundamental than space and time. Yeah.
JUSTIN CURRY: That's for the rest of your life to think about.
[LAUGHTER]
CURRAN KELLEHER: So I'm finished. We have 10 minutes left. I think I'll hand it back to Justin so he can sort of wrap it up.
JUSTIN CURRY: Yeah, great. I really wanted to pull this into the discussion we were having earlier about, what is information? What is meaning? And I want to present you actually with a problem that Curran and I tackled with a group of other people during one of these New England Complex Systems institute intensive week-long seminars that they run in January.
And the fundamental idea of how do we measure information-- how do we measure the content of, say, an image, right? The information content of an image. And why is it that we say that there is more information in an image-- and let's just say we're feeding in to a program things like this. But then, what's inside of it might be something like this.
Now, why do we say that this, in some ways, is more meaningful than just having a bunch of kind of seething dog barf like this? And although I love Jackson Pollock, why do we say that there's maybe less meaning in these lines than when we had the Sierpinski triangle drawing? And it's really a fundamental problem that still people are working on and doing research on.
It involves the way that we can measure information. And one possible measure of information is known as information entropy.
AUDIENCE: Would it be like kind of a randomness?
JUSTIN CURRY: Could it be something about randomness? Well, that's actually fundamentally what a lot of information theory is about, is probabilities, and to what extent do you expect the outcome, right? And it's interesting that one mathematician named Claude Shannon, back in, I think, the '50s, basically, started thinking about this problem and closed a lot of the major problems out in one publication called the Mathematical Theory of Communication. Because he was trying to understand, what does it mean when we're saying something over the phone, and it's going down a transmission line, and there's all this noise from the outside world?
How do we still extract meaning from those pulses in that electrical cable? And how do we get sounds out of that? And what's the information content there?
And if you actually want a rigorous definition of it, if you're talking about one state we can call h of x, and we take the probability of what we're expecting times the log about probability. I can't really derive all this. And then when you're talking about an ensemble of things, you would just kind of sum over this, and this would become a big H. But this is the field of information theory.
But the problem is, is that, fundamentally, it's a question of, if I were to take this image and translate it into a bunch of bits-- 0s and 1s-- how many 0s and 1s would you need for this versus--
AUDIENCE: Maybe you could-- in a picture, you may need more [INAUDIBLE] little abstract you need less.
JUSTIN CURRY: Exactly. So Latief said, so maybe in a picture like this you need more, but when you abstract from it you need less. And this is fundamentally the difference between information entropy and kind of algorithmic entropy. And a really simple problem to think about is, what takes more-- if you were to write a program to give you a number-- let's just pick a number essentially at random and say it's three billion digits long. It's 71743-- so on, so on, dot, dot dot. Since this would be a fundamentally random number by definition, the shortest program which I could write to produce this number would be print this number.
But let's take a different number. What about 3.141592-- anybody here want to show off the number of digits of pi they know, feel free. I had a friend who was actually failing a geometry class, and on Pi Day gave an extra credit point to every digits of pi you could recite. So the hour before class he remembered 150 digits of pi and immediately brought us grade up to an A.
But if you wanted to have a computer spit out pi, it would be stupid to first calculate it out to a million digits, and then write, print, that. But instead, we've got all sorts of different methods for calculating pi. And some of them involve-- well, you could solve it using trigonometric formulas, or you could use all these really beautiful series and approach a digit of it when you have the alternating sum of-- well, for example, if you have-- plus one ninth, plus-- So if you're just taking the sum 1 over n squared, this is pi squared over 6.
So in terms of algorithmic information, it's almost something is more meaningful when it takes fewer lines to describe it. And then that's the argument here, is that if you want to code0 this image into 0s and 1s, this image would take roughly the same number of 0s and 1s as just a bunch of gibberish. And that shows kind of a failure of information entropy. But the fact that we can write a program, like Curran showed you, in just a short few lines that produces this very quickly, shows that it's algorithmic information if you're going to write a program is actually much more meaningful-- It's not algorithmic entropy, sorry-- complexity.
And these are some rigorous mathematical tools which we're using in thinking about what meaning is and what information is. And I really hope I've kind of convinced you that a lot of complex phenomenon can come out of very simple things. Yes, Latief.
AUDIENCE: What if we look at numbers in general, like real numbers.
JUSTIN CURRY: Yes.
AUDIENCE: Because when you give a name to a number--
JUSTIN CURRY: All right, give a name to a number.
AUDIENCE: You only have that so names to give that one number, so you're sort of mixing together different symbols and everything. But even though, given an infinite amount of symbols, you can only give each one a bunch of numbers from one, two, three, four, five, but what you'll end up an infinite amount of numbers, but they match up to integers or something. But you're trying to map to the reals, and there's more reals than the integers, so you have numbers that you cannot even say what they are.
JUSTIN CURRY: So--
AUDIENCE: Does that have something to do with the algorithmic complexity? Because some numbers you can't say what they are. They're, like, not computable.
JUSTIN CURRY: So I mean what you said does have relation to this, but it's actually pretty much struck on one of the most fundamental theorems and paradoxes in the past 30 or 40 years. And I can give you some more information on it, but it's called the Lowenheim-Skolem theorem. And it's the idea that-- and it's really an idea on logic. And fundamentally, the paradox which you just said, shows how if we really want a theory of meaning, if we want to be able to point out every real number but we can only do so in a finite way, how do we know that we actually mean every real number, even though we can do it only in a countable way.
AUDIENCE: What did he say?
JUSTIN CURRY: Sorry. So Latief was asking about when you're specifying real numbers-- so let's take the continuous line from 0 to 1. I know Latief doesn't think this line is continuous, but it is. We only have a certain number of-- well, here, I'll take it to 2, so I can specify here's 1. So for example, the square root of 2-- 1.41-- irrational. Infinite digits-- it does not repeat, so there's no real short way. But we only have a finite number of symbols, a countable number of symbols, in fact, using integers to name this number.
So how can we ever say square root of 2, when we only have or have a countable number of integers to start spelling it out? All we can ever do is approximate the square root of 2. I could give you a score of 2 out to a billion, billion digits, and I still want to be giving you the square root of 2. But then that relates to a really fundamental paradox, which I can't really talk about, because it's just kind of way out over the top.
But suppose you had something like the way we name square root of 2 using mathematics is as whatever x satisfies this equation. And this is really the best we can do. But the idea that you're striking upon, it really is a fundamental one, and it shouldn't be trifled with. But are there any more questions, because I think I'm running desperately over time? And I'm encouraging you guys to think of these problems. Yes.
AUDIENCE: I'm sort of thinking, when you have a Euclean space, it's all continuous and everything.
JUSTIN CURRY: Right.
AUDIENCE: Could you think of consciousness in a similar way? To us it looks continuous, but is it really continuous?
JUSTIN CURRY: So is consciousness continuous?
AUDIENCE: Yeah.
JUSTIN CURRY: So that's a good question, because to what extent do we reduce consciousness just to discrete firings of neurons?
AUDIENCE: As opposed to the subjective feeling that it feels continuous. But just because it feels like something does not mean it is that something.
JUSTIN CURRY: So that's actually a very good idea, because--
AUDIENCE: What did he say?
JUSTIN CURRY: He said, just like consciousness, we feel like it's continuous, but suppose it's not really continuous. One thing to think about is, I think, the refresh rate on our eyes is something like 200 frames per second. And that's really why we think things are continuous. But when we start whirling hand really fast in front of us, we only see it at certain bits, right? But it's kind of from the continuity of our experience-- the fact that it happens so fast that we actually say that we approximate saying that it took all the positions between.
So it's kind of lazy. Yeah. Well, it's the best we can do.
AUDIENCE: It's not good enough.
JUSTIN CURRY: It's not good enough. All right. Fair enough. So I encourage you guys-- it might make the typographical number theory chapter make more sense if you glance over the prepositional calculus. But really focus on the typographical number theory chapter, and then start processing that handout for chapter 10 that I gave you. And hopefully in about two lectures' time or so, we can make sense more of what Godel's achievements were, and maybe try to connect a lot of these ideas. Excellent class, guys.