Flash and JavaScript are required for this feature.
Download the video from Internet Archive.
Description: Class 1 reviews two classic readings (by Solow and Romer) in economic growth theory, turns to a discussion of the innovation-related basis for growth in the 90’s, and notes through NSF data the effect of the economics of globalization and the comparative efforts of other competitor nations. Two elements of direct innovation policy will be introduced: R&D funding, (including the physical science R&D funding challenge) and science and technology education levels, with international comparisons. An investment firm paper will suggest how businesses look at these innovation issues. Part two of two.
Instructor: William Bonvillian
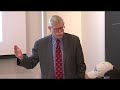
Class 1, Part 2: Economic G...
The following content is provided under a Creative Commons license. Your support will help MIT OpenCourseWare continue to offer high-quality educational resources for free. To make a donation or view additional materials from hundreds of MIT courses, visit MIT OpenCourseWare at ocw.mit.edu.
WILLIAM BONVILLIAN: So Matt was putting a question on the table for us. So why don't you go and lay that out. And we'll get to the next--
AUDIENCE: This idea of how you distribute your talents across R&D sectors. The economic model that stands now is treat technology as [INAUDIBLE] a box and say this. Put this much capital into technology, and you'll get this kind of growth, where it doesn't consider how you distribute your capital within that.
WILLIAM BONVILLIAN: I'll just give you an example because you're right in identifying the problem. About seven or eight years ago, there were a whole series of major studies of climate and how are we going to develop the necessary energy and related technologies that were going to be needed to tackle the problem.
And interestingly, all of those studies got to this moment where they were going to talk about the technologies. And they all basically read the same way. They all said, yes, we're going to need a lot of technologies. And then we'll spend a lot of money on them. We'll spend a lot of money doing research and development, was their conclusion. And then we need to spend this much money on research and development. Then we need to spend this much. But we'll spend a lot of money on research and development.
There was never an attempt to get inside that black box, as you well put it, Matt, of how that innovation system is actually organized to optimize the results. That is our problem. That is the problem of this class, getting inside that black box and figuring out how these innovation systems actually work and how you can optimize the organization of them. And we're going to look at a bunch of models and a bunch of problems, exactly along those lines. But if we do nothing else, you're going to get deep inside that black box and, I think, start to understand some of the elements you've got to think about when you design your way out of it.
Does that help? Because you're right. It's been a classic problem in science and tech policy that it hasn't really faced the innovation organization problems. It hasn't understood how important they are. But I think they're really key. And we really jump into that in the next class.
All right. So we're now at our third great growth economist, Dale Jorgenson, who teaches up the street at Harvard. And Jorgenson I put in here basically because he improves the model. He takes a look at the 1990s IT boom, that period of rapid growth, and shows that that is driven by technological and related innovation. And the resurgence in the US economy in that '93 to 2001 period really outran all expectations.
And his view is that there is the rapid decline in IT prices for a technology that inherently increases productivity in important ways and costs ever less. That's a huge driver and was key to the surge in the 1990s growth. And he argues that the core technology in the IT wave was semiconductors.
So let me just walk through this. You all probably know a fair amount of the semiconductor history. Bell Labs in 1947 with Bardeen, Brattain, and Shockley, they developed the transistor from semiconductor materials. And it's an electrical switching device. It enables essentially the whole follow on of digital technology innovation. It's that digital form that's key.
And then following on that comes the integrated circuit. And that really evolves in 1958. And it's developed in two different sites, two different great groups frankly. Jack Kilby was the leader of a team at Texas Instruments. Robert Noyce is the leader of a team at Fairchild Semiconductor. And Fairchild later morphs into Intel. And they develop the integrated circuit.
Gordon Moore, who's working with Noyce at Fairchild Semiconductor, watching what's going on, he develops Moore's law, which is a good way of describing what Jorgenson is onto. The integrated circuit, every two years, is going to double the number of transistors per chip. And the cost per transistor is going to decline by half in that same two years-- and sometimes, it's 18 months-- kind of time period.
So in other words, you have much greater capability at ever lower cost. That's the phenomena that's at the core of this innovation wave. And that is often what's happening. You'll have a core technology with a declining cost base that gets evermore capable at a lower cost. So you introduce, in effect, a deflationary factor into your technology advanced process.
So in '68, Noyce, Moore, and Andy Grove found Intel. And they move over to microprocessors or logic chips, microchips, which is a bundle of transistors playing out this Moore's law theory. So Jorgenson looks at semiconductors as the core technology advance. And under Moore's law, it's coming down by half every two years in cost. Communications equipment cost is also coming down driven by, in large part, cheaper semiconductors.
So all kinds of transmission technologies are occurring at this time period. I've listed some of them there that are coming down in price. And the result is a technology that's creating major new productivity gains in the economy. In other words, you're producing more for less with less labor input. Therefore, you're creating a real gain, which amounts to a new real wealth in the society, which you can distribute. That's the pattern here. And the result is a growth resurgence.
So there's price declines in computing and communications equipment, computing equipment. Between '90 and 1995, Jorgenson traces a 15%-a-year price decline, between '95 and 2000, a 32% decline per year. In other words, this thing is costing evermore capable and costing a lot less in relatively short time periods.
Now, the outlier here is software. And this is how Bill Gates gets rich because the decline in price in software is only 1.6% between '90 and '95 and only 2.4% between '95 and 2000. So in other words, you can still charge a premium for your software even if the hard technology cost is coming down significantly. So that's why Gates and Microsoft get bigger than IBM because IBM bet on the wrong category.
And we still haven't tackled this cost of software problem. It's still too much of an art form frankly. But there's big growth in the '90s in this area, much higher than any other kind of capital good. And it becomes pervasive in the economy. It's in homes. And it's in every business. And it's in government.
And the productivity numbers. From 1945 to 1973, the productivity growth rate in the US is in the 2% range. And then from '73 to '93, productivity rate falls much lower into the 1% range. And then from '95 to 2000, productivity rate is 3.5%. And economic growth, in turn, is 4.2%. These are astonishing numbers. Historical US growth rate is 3%. In that '73 to '93 period, it falls down to 2%. Productivity falls down to the 1% range.
That's a grim period. That is a grim period in the US. And getting out of that grim period really felt pretty amazing. Guess what? Our current growth rate is in the 2% range. And our current productivity rate is in the 1% range. It doesn't feel robust and dynamic. A lot of people are getting left behind.
What we need? New innovation-based growth wave. That's what Jorgenson is writing about. That's what turned these numbers around in the 1990s. And again, I want you to understand the relationship between growth and productivity gains. Again, productivity gains are what create that real gain in society that you can distribute. And they get driven by technological advance. Productivity gains get driven predominantly by technological advance.
So that's Jorgenson's picture. He essentially proves the model. He basically shows, by looking at a period of a big innovation wave, that Solow has got it right. It's technological-based innovation that's driving that amazing period of economic growth in the 1990s. So questions about this.
AUDIENCE: For that dip in the '70s to '90s, is that mostly attributed to policy or demographic change? What was going on there that was resulting in lower innovation?
WILLIAM BONVILLIAN: There's a number of things going on. It's not so much demographics change in that period of time. Now, we've got much more significant demographics issues. And the cost of an aging demographic because you're going to have to pay for me. And I'm going to take a lot of your real wealth. It's an intergenerational transfer now. We didn't really have much of that going on in that time period. It was the ascendancy of a baby boom, which in some ways is the opposite.
But in that period, that's when the competition with Japan hits. And we'll talk more about this. But in the post World War II period, the US organizes its innovation system. And it organizes its innovation system around leading these innovation waves. And it ends up leading almost all of them in that late-20th-century, second-half-of-the-20th-century period. And it gets very rich because it's getting the first mover advantage of these innovation waves. So we create the richest nation on Earth.
And then it misses one. And we'll talk more about this in class 3. But Japan figures out quality manufacturing. It's an innovation wave. It's a whole new way of doing production. And out of that, Japan is able to capture very large parts of consumer electronics and the auto sector, which had been core sectors in the US economy. So a fair amount of what's going on between '73 and '93 is that the US misses an innovation wave.
Now, interestingly, then what happens? Then we have this boom time. What did we do? We brought on another innovation wave. This is the IT wave. That's what Jorgenson is telling us. And fascinatingly, Japan missed it. So there's a lot of lessons here that we'll talk about when we get to the manufacturing class. But go ahead, Max.
AUDIENCE: [INAUDIBLE] I mean, if you've got companies like Sony that did very well.
WILLIAM BONVILLIAN: I'll describe for you, Matt-- let me interrupt since you mentioned Sony. So I'm in Japan in January of 2006 speaking at a big conference organized by the US National Academies and similar organizations in Japan. And the meeting is on innovation systems actually. And the headline in the major Japanese newspaper-- and there's a major Japanese English language newspaper. The headline is-- bold headline across the front-- Apple Sells 14 Million iPods, semicolon, where is Sony, question mark, question mark.
Apple took back a large part of that consumer innovation wave. Snatched it because of defeat at the hands of great companies like Sony. So the US was-- US quote because Apple doesn't make anything here-- was able to take back leadership of an important because of its leadership of a computing revolution. In effect, it adds a computing revolution onto what that had been consumer electronics and then leads in that territory. And that's what's playing out in this time period of the innovation wave. But we'll dive into this when we get to the manufacturing class.
So anything else on Jorgenson? What do you think? Is he right? Is this the right picture? I think the economic evidence is pretty overwhelming on this one. It looks really pretty clear what happened. And to this model of a core invention, apps pile on it, semiconductors, apps pile around it like the internet. And then it becomes innovation. And then it becomes an innovation wave.
And then it spreads throughout the economy. And then it creates big productivity gains. And then you can translate those productivity gains into a real gain in your society, which means a real new wealth in your society which you can distribute. That looks very clear that that happens. So a major issue in the class is how do you how do you do these? Can we do these? Can we speed the pace? I mean, typically, they happen about every 40 or 50 years. Could you reduce the interim period?
One way of looking at what's going on now is we're just waiting for a wave. It's like we're surfers paddling around waiting for that wave to kind of take us. And I don't know what that wave is going to be. But it could be very interesting because we seem to do these about every 40 or 50 years. But then the question is, are we going to lead it?
So if the next big wave worldwide is energy, and there's certainly a case that it might be, are we going to have a first-mover advantage in a lot of these technologies and get some of the gains? Or not? I think that's a pretty open question.
AUDIENCE: Chime in.
WILLIAM BONVILLIAN: Martin, go ahead.
AUDIENCE: Yeah, so in general, that's the classic thing of, if we miss the next wave, your economy will plummet too. And that's probably what happened in Japan. The big concern, though, is like most like Schwinger, who's the nuclear scientist in the 50's, 60's, talked about how, if we do nuclear, it wouldn't be possible in the US, It would probably have to be somewhere in Asia because we're just kind of close-minded to it.
And then if you look into recent examples, like Bill Gates funded a fission company that's doing a new kind of reactor. But the policy just so hard here that they're just doing it in China because it's faster. And I think Romer also talks about charter cities where you have less rules. So it's easier to innovate quickly. So that's another thing. There's like this saying where, if you outlaw innovation, only outlaws will evolve. If you make it really hard to innovate, people are just going to go somewhere else.
WILLIAM BONVILLIAN: I think those are important points, Martin. And this is a legacy sector problem. In other words, the US-- and we'll talk about this a lot when we get to energy. And we'll read about it. But the US is pretty good at creating these kind of new frontier territories, standing up these new areas. We're not good at bringing innovation back into established sectors. We'd just rather do the next big thing. And that's not bad. But when a lot of your social problems are tied to the legacy sectors, then it becomes a big disadvantage.
Now, that's not to say that countries in general are good at innovating in legacy sectors. They're not. There's a problem for everybody. But I think we're particularly bad at it. And think of what the big societal problems are. Energy efficiency and moving to a new generation of energy technologies. We've got to figure out how to do that. But that means learning how to innovate in a legacy sector. We've got a terrible problem with health care delivery, terrible.
We do the new thing. We'll do biotech. We'll create the new frontier. We just don't go back and fix the health care delivery system because it's tough. We'd rather do biotech. So a lot of our societal problems are tied up in this stuff. And as we'll talk about in a couple of weeks, manufacturing has played a critical role in creating a very deep inequality problem in society, the failure of that sector.
And again, we're not good at going back and bringing innovation, i.e. the next generation of advanced manufacturing, into an established legacy sector. So this legacy sector problem actually turns out to be a big one. And that'll be one of the themes of the class. I'm stealing my own thunder for a few weeks now. But you get an advance snapshot. Chloe?
AUDIENCE: Are we at a new point in our history of innovation because we have too many next big things that we're having problems following through creating an innovation wave? We have health care and space and energy and nuclear.
WILLIAM BONVILLIAN: Yeah. I don't think it's bad to have a big menu to get waves out of. I think that's fine. And the other thing I should say is not all waves are big waves. In other words, waves are of different size here. Biotech is not a big a wave as an IT sector. But it sure is an important wave.
And you don't want to miss it. So we're going to have different waves of different sizes. So having a lot of candidates for waves, I think, is actually a pretty important thing. Because it's hard to predict where the big innovation waves will actually go. Does that answer that? Any other thoughts? All right, let's do this Merrill Lynch piece.
So here's the question that this-- this is a little Merrill Lynch report that is sent just before 9/11, interestingly, when the markets went bust. This was done on September 4, 2001. And disaster is only days ahead. But this is that they're riding the IT innovation wave. And they're trying to assess what innovation waves are and how their investors can get rich off of them. And in a capitalist economy, that's not an unimportant task.
So how do investors look at potential technology breakthroughs? Do they believe that they drive growth? So what does the Merrill report out to its investors, tell its investors? Well, they say, yes. Innovation drives growth so you want to get on this. You really want to get on these innovation waves.
And then Norman Piore, who I think is related to MIT's Michael Piore, who's a wonderful professor of entrepreneurship and technology advances at Sloan, has done important work on a lot of things, including DARPA and including manufacturing. So I think Norman and Michael are related. But I haven't asked him. But Norman Piore, who's the chief economist for Merrill Lynch at the time, he said, yes, innovation-based growth drives the economy. And by the way, it drives the stock market.
And then he goes on to say-- and where he gets this, I don't know. But he says, it takes 28 years for widespread acceptance of a new technology. That's-- is it always 28 years? Maybe it's 27. I don't know. Then it takes 56 years for rapid growth to evolve. And then it takes 112 years measured from here for technological maturity to occur. And then after that, growth in that sector is going to resemble growth in population rates.
Well, I don't know where he gets the 28 years, 56 years, 112 years. I don't know where that comes from. But this is the chief economist of Merrill Lynch saying, yes, this is how it works. This is how it happens. That's interesting. It's not just an obscure economist conjury of growth wave. This is advice going out from, then, the largest stock, broad-based investment company in the US.
Then they hit on, what's the wave we're going to hit? So they think nano. That's the one, nanotechnology. So they describe an interesting pattern here, which I think is actually very important. It's really, I think, very perceptive. I don't know where the 28 year comes from although the time frames are roughly right. But this, I think, is really intriguing. And I want you to think about this.
So they say, let's look at nanotechnology. And as you know, that's fabrication of the mark of the scale. And first, for a technology to evolve and get into range of being innovation and maybe an innovation wave, first, there's got to be a vision. Somebody has to present a vision of what this technology could accomplish. And they argued that the first vision about nanotechnology was from physicist Richard Feynman, a famous physicist who went to school here, of course, and was in the Manhattan Project and then unfortunately taught at an obscure California school called Caltech.
But Feynman, in a 1959 piece, argued, in the physics community, there's plenty of room at the bottom. In other words, at the really small scales were quantum effects can occur, some really interesting things can happen. And that's the nano scale. So he gives a vision that we could do some really interesting stuff when you're operating outside of Newtonian physics at a smaller scale.
So the second piece that has to occur they refer to as enablers. So there's got to be, in effect, tool sets and instruments that enable this vision to get played out. And the key tool set in that time period was when IBM did the scanning tunneling electron microscope. And you probably don't remember this. But there was a moment when IBM, before there was much of an internet, distributed photographs of stacks of molecules spelling out the IBM logo and to civilians like me, I said, oh, that looks very nice.
But to scientists seeing an ability to structure molecules in that kind of way was just absolutely amazing. So the scanning tunneling electron microscope is not only an observing system. It's a device by which you can move molecules around. So it becomes a huge capability in allowing measurement and manipulation in nanoscale systems. So that's when this article was written. That was 20 years before.
The third piece that this Merrill Lynch piece talks about, which I also think is really important, is this is straight out of Romer. You've got to create research mass. In other words, you got to put talent on the problem. This is human capital engaged in research. So Eric Drexler, from MIT, in a 1981 journal article, begins to describe the kind of physics and possibilities in nanotechnology. That's the first one.
By 2000, there are 1,800 journal articles on nano. And by the way, that's a similar phenomena to the number of articles starting to write about the internet in the 1990s. In other words, this journal article total signals research mass. It signals prospectors. It signals human capital engaged in research. So these are, I think, really interesting conceptual points here.
And you have to go through these to get to your innovation slash innovation wave. You need the vision. You need the enabling tools. And then you need talent on the task, this research mass. And you've got to go through all three steps to get there. That's interesting.
And then they come to, how are we going to tell our investors to get rich off this? Because we've gone through the three stages for nano. We've got 1,800 journal articles the previous year. We've got research mass. How are we going to get rich? And then they issue this warning. Although the futuristic market is fascinating, it is not inevitable.
So just because you're approaching the future, it doesn't mean you're always going to get rich. So how are you going to get rich? They acknowledge that nanotechnology in 2001 is starting to get close to commercial markets. And then they review key markets, which we would view as hilariously short term. So zero to two years, short term, zero to five years, mid-term. Five-plus years is long term.
Now, remember Norman Piore? It takes 28 years to do this and takes 56 years to do this. It takes 112 years to do this. Those are long periods of time. These guys are talking about trying to get rich in what we would view as extremely short-term periods. And they give you a good perspective as to how far out investors are even willing to look. And they argued that the keys to nanotechnology are manufacturing and communication. If you can't build it in volume, then there's not much you can do with it.
So they look at a series of opportunities with that idea behind. They look at opportunity number 1-- instrumentation. And they note that, as a new technology is advancing along, the first class of winners are the tool makers. So we'll go back to our California Gold Rush in 1849. The first people to get really rich in California are those that are supplying those miners that are headed off to the gold fields. The tool makers tend to be an early class.
So for heaven's sakes, let's invest in the tool makers because they're critical to everybody else. Everybody is going to need their stuff. Let's invest in that stuff. Then they look at semiconductors. And they note that, within 10 years, if you're going to stay on Moore's law, you're going to have to get deep into nanotechnology, which, of course, turns out to be the case. But that could take 10 years. So forget that. They're not going to advise any investors to get into that business. Instead, they really want to focus on stuff that can evolve in two years or less.
So if Norman is right and technology development takes a really long time measured in decades and Merrill is only willing to invest for what it refers to as the short-term zero to two years, there is a big disconnect in our ability to stand up technologies. We're just not operating at a plausible frame. And who is going to fund the rest of it? I don't think Norman's 28 years is necessarily right. But it's a long time. And who is going to fund that? Who's going to carry this stuff for that extended period if Merrill and the boys are only willing to do a two-year timetable?
This is a big gap in the innovation system. And when we were talking a few minutes ago about the problems we've got in standing up hard technologies, investment at scale, if it's only going to focus on a two-year time frame-- in other words, you've got to get to production in the short term to really have this investable, to work as an investment, so you're not going to want to invest in technologies that are more than two years out from production-- then you're taking an enormous amount of interesting things off the table here. So it's a really big structural problem.
The answer that the US has come up with historically is that the federal government will play a long-term role. The federal government will provide the long-term patient capital. But that's not a perfect model either because of what we'll talk about next week, this valley of death problem. So you following me?
So this is a classic kind of innovation system problem. So when you're thinking about innovation systems, you're going to have to think about the actors in that system, the handoffs between the actors, and then the time frames that the actor is going to need support. So this is another way of helping us think about how to look at innovation systems.
Any questions flow from this? Because this is a big challenge here. The venture capital time frame is basically organized for everything except biotech when looking at technologies that are no more than a couple of years out from production. So the US created this amazing venture capital system, which, in many ways, is the envy of the world. And it works very nicely for IT. And it works for very different reasons that we'll talk about for biotech.
But it's not working in these hard technology sectors because the timetables are just too long and the risks are too high and the uncertainties are too great. So this is a big gap in the innovation system. Merrill Lynch doesn't look at it as a gap in the innovation system. But that's really what they're showing us exists.
And then the other important thing to remember about what they present us with is I think this very perceptive notion that you need to go through these three stages. You need to move a technology from invention and discovery to innovation-- vision, the enabling technologies, and then the research mass, the human capital engaged in research, the talent base.
AUDIENCE: So are innovation systems, like Bell Labs, just unsustainable? Or why haven't we seen anything like that?
WILLIAM BONVILLIAN: Yeah, I mean, we'll deal with this a bit next week. And you should, Beth, bring me back to this next week for sure. The short story-- and we'll spend a little more time on this. Bell Labs was tied and was able to have very patient long-term supportive technology because it was tied to a government-guaranteed monopoly model.
And when we broke up AT&T and the segments that survived were placed in a highly competitive situation with one another plus an IT revolution, which created an entirely different communication system around the internet, was descending, then Bell Labs' ability to sustain a model of long-term patient investment in breakthrough technology advance, it's gone.
And by and large, with some exceptions, the model of the large industry-supported, basic and applied research laboratory has largely gone. There's still some pieces left. IBM, although that's under a lot of economic pressure as it tries to shift to a service-based business and wonders why it's doing hard technology research. That's a remaining piece. But it's not what it was a decade ago in terms of its basic research support.
So there's few of these left. And the model of incredible global competition has really eroded the ability of a company to take a long-term risk over highly hypothetical research advances that are very high risk. It's just really eroded this. So what's been happening?
So universities are increasingly being asked to take this job on. So universities have gone from a 19th-century model of education as their dominant driver to a mid-20th-century model of adding research to education and merging those to, in recent decades, playing an economic role, which they never played before, and trying to figure out how to play that role.
Because, in effect, they're the surrogate piece to substitute for the Bell Labs model to sustaining longer-term, higher-risk technology development. And they're just beginning to figure out how to do this. So the engine at MIT is a really interesting model, which we'll talk about. And if anybody has any ties over the engine, we probably ought to go over there and take a look at what they're up to, wander down the street, and talk to them.
AUDIENCE: Just to clarify--
WILLIAM BONVILLIAN: Go ahead, Max. Go ahead.
AUDIENCE: What is the engine?
WILLIAM BONVILLIAN: We'll talk about it. But MIT is attempting to create a place for startups that are right up against this wall of not getting venture support doing primarily hard technologies where they can live and have access to advanced equipment, advanced technologies, and a lot of know-how. So in effect, it's substituting a place for the kind of stuff that they had to carry out with venture funding before.
So if you throw a group of them together, they have shared assets-- in effect, Creative Commons-- rely on this campus but also secondary nodes like Lincoln Labs, like some companies that are interested in this model to help them scale up their technologies. So there's a lot of incubators in this neighborhood. We have eight incubators very close to MIT. And they're very interesting. And some of them are terrific.
The incubator tends to focus on getting your business plan together and kind of perfecting your initial prototype. This is an attempt to do something that goes to much later stages to help you do the advanced prototype, late-stage development, demonstration, test bed, and maybe even pilot production, which is typically what you get venture funding for. But the timetables don't work for venture funding. Maybe by substituting space for venture capital, you could create a different kind of model.
So MIT is busy taking this amazing adventure trying to do this. And that's just a classic example of how university is going to have to wrestle with their new economic role to get around this Merrill Lynch-identified problem for us.
AUDIENCE: Also, there's sufficient capital--
WILLIAM BONVILLIAN: Yes. Right. And part of this-- pure space doesn't always solve the problem. You're going to need some bridge funding. So part of this is raising some money. And could you raise-- the return on venture capital is designed to be high. And it is designed to be pretty short term to get your money back in a pretty reasonable time period in these venture funds.
This is not going to happen with these hard technologies, a number of which will be coming out of Martha's world of energy technology development. Is there a community out there that's prepared to tolerate a much lower-level-return, higher-risk, and long-term focus just for societal well-being. Stefania?
AUDIENCE: Estefania.
WILLIAM BONVILLIAN: Estefania.
AUDIENCE: There you go.
WILLIAM BONVILLIAN: All right. You're going to get me to do this right. I promise.
AUDIENCE: I think that's precisely why it's so interesting to study the nonprofit development model and why it's important to understand how to rebrand science and technology innovation processes and systems in particular because nonprofits, for a very long time, and especially community-led and local nonprofits, have understood exactly the consequences of resource scarcity no matter how good their ideas are.
And that's why precisely I appreciate it on her articulation of the ways in which-- was it the Manhattan Project in Kansas, the great teams model?
WILLIAM BONVILLIAN: Great groups, yes.
AUDIENCE: This model that we'll talk about later. But I do think it's important to have a sort of critical literacy of funding models in the way that does impact the kind of research that you can pursue and also how that funding model will impact the ways in which you'll have to construct your narrative of success, whether that's through a publication or the product that you actually end up producing.
Because it turns out that there's different kinds of venture models. And I'm sure that MIT is very cognizant of the ways in which donor-specified funding plays a role in shaping what you can and cannot pursue as a researcher.
WILLIAM BONVILLIAN: Yeah. And interestingly, exactly as you say, we're starting to play around with different mechanisms including a number of nonprofit mechanisms, that would help us to kind of fill this gap in the innovation system. And in effect, Martin, back to your point, enable us to have a lot more kitchens.
All right. Let me see if I can draw some conclusions here. This class has talked about two direct innovation factors, things that I would argue that you can't do without. So if Solow, which its growth is driven by technological and related innovation, is right-- it's responsible for 2/3 of historic US economic growth-- then having an R&D system is a pretty critical pillar. And you need to invest in that system. So that's factor number 1.
Let's look at some of the numbers. And this is-- one of the readings this week was from NSF indicators. And NSF indicators comes out every other year. It is the great collection of data on the US innovation system with lots of comparative international assessments as well. And it's just a great tool set for you as you look at innovation systems and need to make comparative analysis.
So don't miss it. I don't want you to read it. Just glance at it. See the kinds of things that they that they can collect data for you on and what those plots look like. And I'm pulling a few of my favorite charts, sometimes innovators but almost always from NSF-developed data.
Federal research funding. So this is federal R&D outlays as a percentage of total federal government discretionary spending between '62 and 2008. And you see that discretionary spending on R&D began to approach it's like 17% in 1965. And then it comes down to well below 10%, and actually barely above 8% by 2010. So that's another way of looking at that same curve. So that blue curve is a slightly different measurement. But that's essentially the decline of federal government support.
But then you see, in the purple, it's an interesting x curve. So you see an increase in industry support for R&D over that same time period. By the way, you can extrapolate this out and the same phenomenon occurs. And this x curve is a very famous curve. So you think, oh, well, it's too bad that government is pulling out of R&D. But isn't it nice that industry is taking over. And great. Problem solved. Because we're essentially at the same percentage of GDP that goes to R&D as we were back in the 1960s. So no problem.
But then you figure out that these two different lines are measuring-- it's apples and oranges. Government supports predominantly research. Industry supports predominantly development. Is there a relationship between research and development? Well, yes, of course, there is a relationship between research and development. To a significant extent, research is going to drive development over an extended period of time.
So then this picture becomes very problematic. In a way, the government investment back here enabled this big industry build-up in development that followed afterwards over decades afterwards. But if you're bringing down your research, over time, that's going to affect your ability to do development.
So this x curve, it's a very problematic curve for the future of US innovation because what you want are two parallel rising lines. You want rising research, which in turn is going to enable rising development. You don't want either or. It doesn't work.
I mean, there's a phenomena here. You have to understand why was this government level so high. Imagine that. 2/3 of R&D is being spent by the federal government here in the 1960s. What's going on? Space race. Cold War. And hot war. So we've got simultaneously a hot war, Cold War, and space race. That does wonders for science investment. And obviously, fortunately, we haven't replicated that, to the extent anyway, that it occurred back in that time period.
That's the x curve. Let me go back to this. This is investment in development, which, as you can look, you can see the x curve there. And that's investment in basic research. So government dominates basic research. The blue industry dominates development. Why percentage of GDP? Is that real? That's the best measure for showing what the societal commitment is to research. How much of your society's wealth are you spending on R&D? That's probably the best measure that shows the societal commitment.
This is where it goes. So health is the blue. And that's been the big expanding area in federal R&D funding. And that doubles starting in 1988. And you can see that take off, whereas the rest is fairly stagnant. Here's another way of looking at that. Federal non-defense research and development trends as a share of GDP-- health now dominates.
Other nations are obviously working hard on building their R&D capability. And you can see that red line of the US kind of stagnating. A lot of other countries aren't following that model, particularly China on the bottom. They understand the need for R&D investment. So these are some ways of looking at that Solow thing. What are we doing on R&D? These are ways of quantifying the investment levels around R&D, both on the governmental side, which tends to dominate research, and the industry side, which tends to dominate development. And the pictures are not ones that we really want to have.
Let's look at our second direct innovation factor, the Romer factor, the talent factor. So if Romer is right, human capital engagement research is the critical input for the follow on technological innovation. It's a prerequisite for running your R&D system. And that's the prospector theory and the talent-based theory of growth. So then talent development becomes another key pillar for looking at the strength of your innovation economy and looking at your innovation capable.
So let's look at some of this. That's natural science and engineering doctorates in selected countries. And you see particularly this incredible rise in degrees coming out of China but a lot of growth in a number of other countries as well and a fair amount of stagnation in the US.
This US innovation depends on the presence of foreign-born scientists and engineers. That's a very important part of our system. And that's how this curve has taken off. So this is very important input into the US innovation system. That's why immigration is such a huge concern for the 400 tech companies that filed the amicus brief for today's argument. This is the annual growth rate on numbers of researchers by country. I mean, the US has the largest research pool by far. But the growth rate is much lower. You see the rising growth rates in a series of Asian economies.
This is world share of natural science and engineering publications. You actually see a decline in world share from the US and, obviously, a huge rise in world share from China, but also countries like South Korea. Significant increases in first university degrees in natural science and engineering in China against the rest of the world. China, rest of the world, right?
They get an innovation-based growth model. That's what they're after. They understand its importance. They are a developing economy that's become an emerging economy that's using an innovation-based growth model for its growth. So these are ways of looking at talent and the strength of your innovation system. And the NSF indicators can tell us a lot about the second pillar, this Paul Romer pillar, this talent pillar.
Now, where did these direct innovation system factors come from, these two? Well, on the direct governmental role, federal government funding of university research is obviously key. And government dominates research investment as we saw. Things like government labs are obviously government dominated. The education and training system, these are Romer-type factors. The federal government dominates higher education support for science and engineering.
Support for industry R&D, that's pretty significant on the government side, particularly through the Defense Department. And sometimes some other agency missions, but DoD is the big one. So this is the government playing a role in direct innovation factors, both education and R&D investment.
But then the private sector plays a significant role in these two direct innovation factors as well. So industry R&D, which as we talked about, it's primarily development. Industry takes things through the engineering prototyping and production stages. The training system is dominated by industry. So that's a Romer-like factor. So you see the division between public sector and private sector roles around these two innovation system pillar. Any questions about this data stuff?
So let's do some wrap-up. Robert Solow, the key to economic growth is technology and related innovation. And for shorthand-- although it's not a fair summary, but in shorthand, we could say that you've got to do R&D. Then we talked about Paul Romer. Behind that technological and related innovation factor is what he calls human capital engaged in research. It's your talent base. But again, it's got to be in the system. It can't be driving cabs. It's gotta be in that system.
And Dale Jorgenson tells us that the key to 1990s growth actually was technological and related innovation, that Solow is absolute right. That's how the economy grew. And we saw the picture that he presented for us of a core technology, semiconductors, applications pile around it, it enters the economy at significant scale and starts to affect many sectors. That enables productivity gains. The productivity gains is a real gain in the society, which can be distributed to improve societal well-being. That's the picture he uses to show how these innovation waves actually operate.
And then from the Merrill Lynch piece that we read, we learn that investors are only prepared to tackle a pretty small slice of that long-term technology development time frame that's needed. They acknowledge that it's long term. They're going to focus on a very short part of it. So you've got automatically a big gap in the US innovation system that's going to have to get wrestled with.
And then the last piece we went through were these direct innovation factors, R&D and talent, and the different roles that both government and private sector play in these two. And we looked at the NSF indicators as a good source for you for terrific data on how to look at innovation, the US innovation systems but also comparative analysis with other nations as well.